top of page
Support Experience Conference 2024
On-Demand Videos
Watch every session from 2024’s in-person conference — including product keynotes, solution deep dives, and panel discussions.
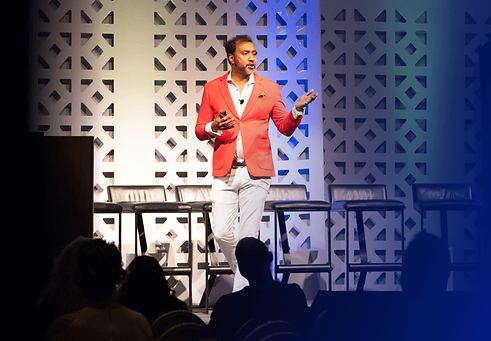
Intro — SX Live 2024
Keynotes - SX Live 2024
Panel Discussions - SX Live 2024
Solution Deep Dives - SX Live 2024
bottom of page